Imagetosketch translation is to learn the mapping between an image and a corresponding human drawn sketch.
Machine can be trained to mimic the human drawing process using a training set of aligned image-sketch pairs.
However, to collect such paired data is quite expensive or even unavailable formany cases since sketches exhibit various level of abstractness and drawing preferences.
Hence, we present an approach for learning an image-to-sketch translation network via unpaired examples.
A translation network, which can translate the representation in image latent space to sketch domain, is trained in unsupervised setting.
ImageToSketch
To prevent the problem of representation shifting in cross-domain translation, a novel cycle+ consistency loss is explored.
Experimental results on sketch recognition and sketch-based image retrieval demonstrate the effectiveness of our approach.
Sketching has a long history in human society that people can draw a few line strokes to record visual world since ancient times.
Defined as sketch synthesis in computer vision, which aims to teach machine to generate sketch from real image just as humans do, has been attracted increasing attentions lately.
Human visual system is so powerful that people can easily draw a sketch to express a complex real-world object just given a glance, whereas it is quite challenging for machine to perform similar ability due to the inherent ambiguities in sketch, e.g., highly abstractness and large appearance variance thus leading to severe cross-domain gap between image and sketch.
ImageToSketch success learning
Recently, due to the success of generative adversarial learning, sketch synthesis could be treated as an image-to-image translation problem.
However, all the prior arts, typically require tens of thousands image-sketch paired training examples to alleviate the above-mentioned difficulties.
Requiring such a large amount of data is notorious since it is labour costly to collect the one-to-one mapping image-sketch paired data.
Therefore, in this paper, we propose an unsupervised image-to-sketch translation network which could be trained only given unpaired image-sketch data.
The problem of unpaired/unsupervised image-to-sketch translation is difficult due to the large cross-domain gap—no paired examples showing how a real image could be transferred to a corresponding human sketch.
ImageToSketch problems
Similar problem has been studied for unpaired image-to-image translation, which has achieved impressive results by using cycle consistency based on coupled GANs.
However, our unpaired image-to-sketch learning task is considered as much harder due to the larger domain gap exists comparing with the image-to-image case.
To solve this problem, an end-to-end network based on variational autoencoder (VAE), and generative adversarial network (GAN)is proposed.
Specifically, we model each domain using VAE to obtain their encoder and decoder, i.e., (Eimage, Dimage) and (Esketch, Dsketch).
Then we attempt to learn a translation network (TranNet) to convert the representation in image domain to sketch domain, i.e., TI→S (image) → sketch, which could be further used to generate a corresponding sketch Dsketch (TI→S (image)).
A novel Cycle+ Consistency is developed to explicitly restrict the representations in two latent spaces for the same input image to be consistent.
Edge of real image is importantly embedded as an additional shape prior for regulating the translation of the representations to prevent representation shifting, hence can enforce a better image-sketch resemblance in appearance.
The contributions of this paper can be summarized as follows:
(1) an unsupervised model based on VAE-GAN is proposed for stroke-level sketch synthesis by using unpaired image-sketch data,
(2) A novel cycle+ consistency loss is designed for regulating the domain-specific representations to be consistent, hence restricting the TranNet to be instance sensitivem
(3) Edge cue is utilized to further constrain the TranNet to learn to encode shape knowledge provided by input image,
(4) Our model is also applicable to generate image from sketch in reverse order.
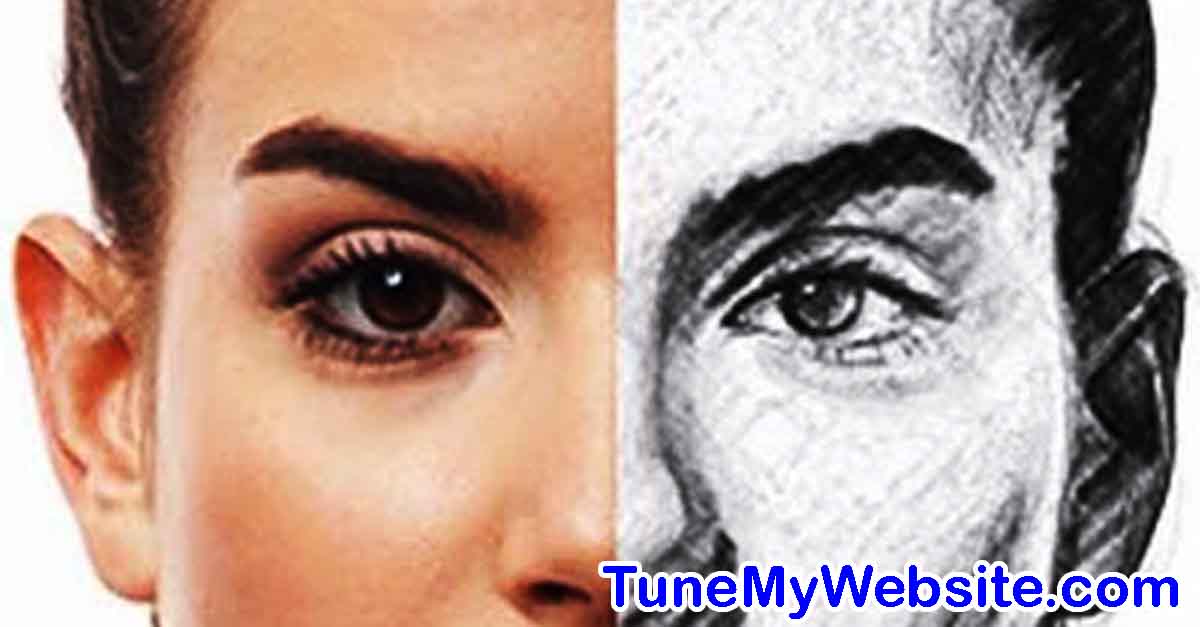